Basic Tips
This lesson preview is part of the The Basics of Prompt Engineering course and can be unlocked immediately with a \newline Pro subscription or a single-time purchase. Already have access to this course? Log in here.
Get unlimited access to The Basics of Prompt Engineering, plus 80+ \newline books, guides and courses with the \newline Pro subscription.
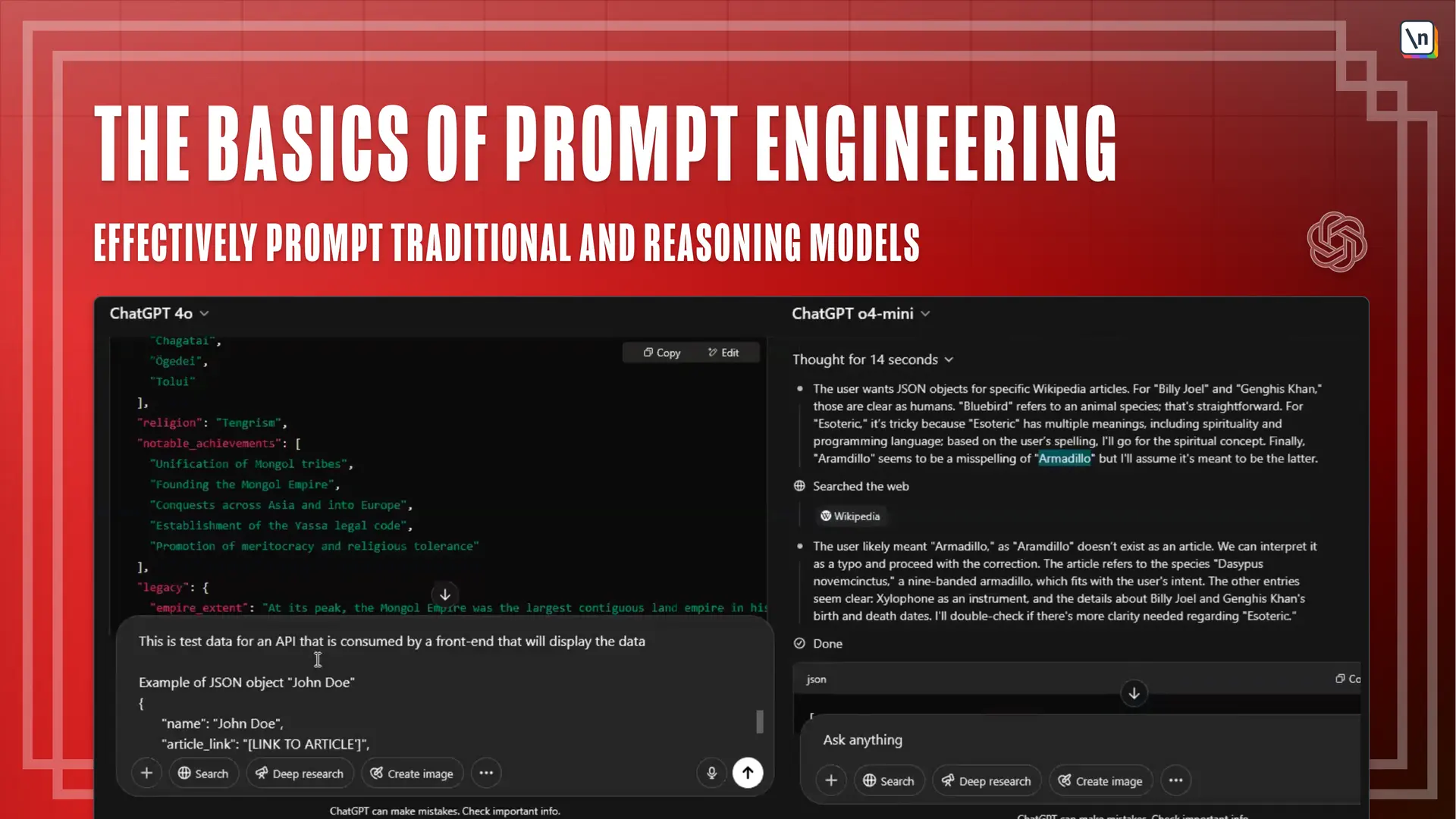
[00:00 - 00:09] Now, I'm going to give some basic kind of overall guidance. This is mostly common sense stuff, but I often see people missing it.
[00:10 - 00:18] This is also stuff that applies no matter what kind of model you're doing. If you take away anything from this session, this is probably what you should take away.
[00:19 - 00:26] You don't always have to make your prompt super complicated. A lot of the times, a simple zero-shot prompt is all you need.
[00:27 - 00:42] And usually a zero-shot prompt is not super expensive. You can try it, and if that doesn't work, you can then refine your prompt and use some of the concepts that we're going to go over today to get a more consistent, more specified result.
[00:43 - 00:55] Another kind of, I guess, relatively straightforward thing to keep in mind is to just be very clear and direct. I know a lot of people, for example, will add please or thank you to their prompts.
[00:56 - 01:12] But that's not really necessary. You might actually have seen this tweet from some ultimate recently, where he estimates the cost of all the additional tokens from people saying, "Please and thank you to be somewhere in the millions over the lifetime of the various chat GPT models."
[01:13 - 01:18] Personally, I generally don't do this. I try to avoid any unnecessary extra bits of language.
[01:19 - 01:28] Obviously, a "Please and thank you" isn't really going to affect anything. The model kind of knows that these are generally not words that need to be taken into the processing step.
[01:29 - 01:48] But yeah, worse than, let's say, words that aren't direct is anything that's ambiguous or anything that's kind of like a passive tone, right? We're talking about verbing the noun versus the verb in is happening to the noun kind of stuff like this.
[01:49 - 01:59] Vague language, direct language. And another thing you can do is obviously, if you're using our LEMs, is do test out different models.
[02:00 - 02:11] Most of them have a free tier that lets you at least play around with it a little bit. There's a lot of benchmarks out there that ostensibly say that one model is better at another model than something.
[02:12 - 02:24] But I think honestly, personal preference is often more important than something being two percentage points better at answering algebra or something, right? Unless you have a really specific use case about hinges on those benchmarks.
[02:25 - 02:38] So yeah, test around, try different problems on different models, see what works best for you. Honestly, have some friends that's whereby clod for one thing, cut GPT for another, and so on and so forth, right?
[02:39 - 02:45] They have different strengths and weaknesses. And people just are going to have different preferences if they test around a few different models.